Deep-learning facilitated microscopy for the dissection of durable resistance to plant disease.
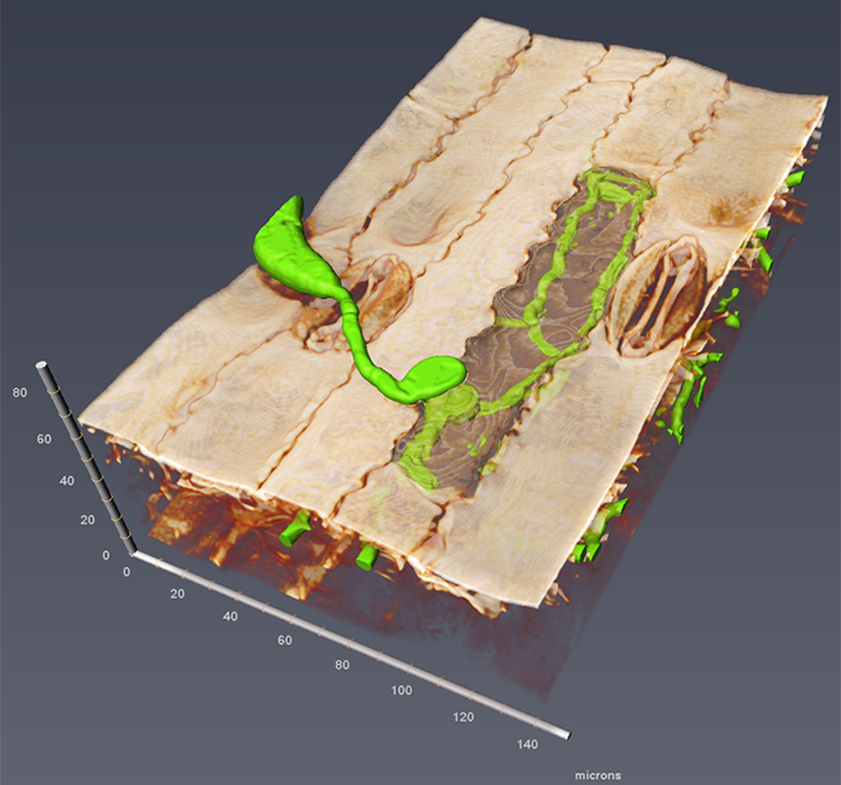
Project Information
deep-learning, pythonProject Status: Complete
Project Region: CAREERS
Submitted By: Anita Schwartz
Project Email: jcaplan@udel.edu
Project Institution: University of Delaware
Anchor Institution: CR-University of Delaware
Mentors: Randall Wisser, Philip Saponaro, Anita Schwartz
Students: Hening Cui
Project Description
Using existing image datasets from different microscopy platforms, we would like to cross validate and, if needed, retrain the deep-learning model (U-Net CNN) used by DeepXScope for 3D segmentation. Cross validation and retraining of the model will be performed using real data as well as virtual data (fungal infection on host tissue is created by computer simulation). With a validated pipeline, DeepXScope will then be applied to available datasets to generate associated output data for downstream analysis and biological interpretation.Background: Plant diseases limit the production of crop plants worldwide. Durable forms of natural resistance (genetically determined) are an environmentally friendly and sustainable solution for disease control, but the biology of durable resistance is poorly understood, particularly at the microscale where the pathogens are visible. To better understand pathogenesis, we developed DeepXScope (https://github.com/drmaize/compvision): a deep-learning facilitated pipeline for segmenting 3D microscopy data and quantifying features of host-pathogen interactions. Working in maize (corn) on a fungus causing prior epidemics, DeepXScope is being used to show how macroscopic disease outcomes arise from microscopic events during pathogenesis.
Additional Resources
Launch Presentation:Wrap Presentation: 6 months
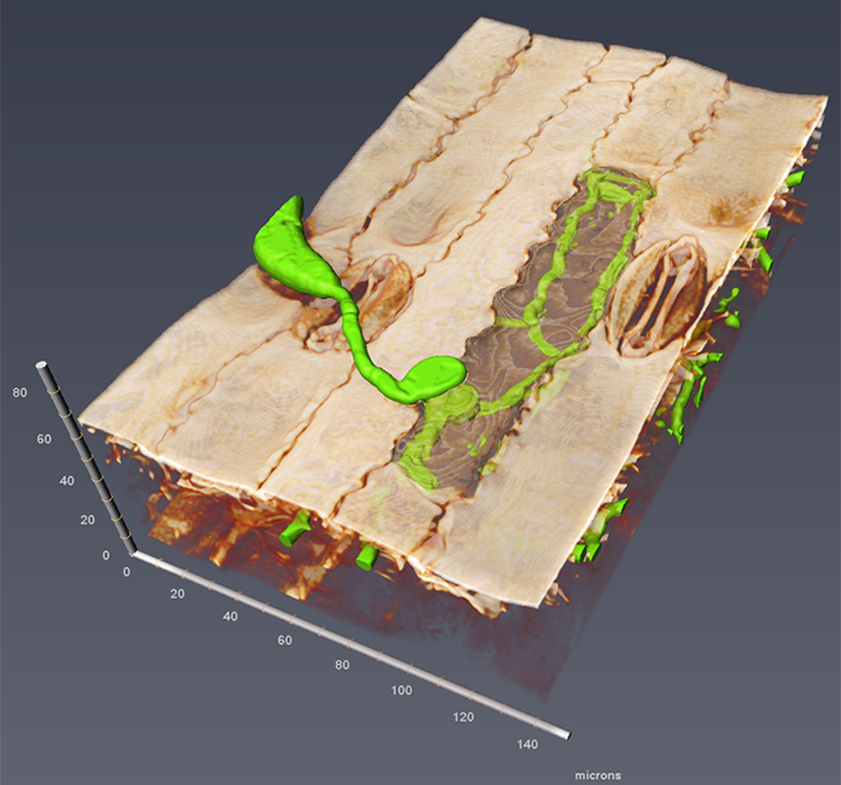
Project Information
deep-learning, pythonProject Status: Complete
Project Region: CAREERS
Submitted By: Anita Schwartz
Project Email: jcaplan@udel.edu
Project Institution: University of Delaware
Anchor Institution: CR-University of Delaware
Mentors: Randall Wisser, Philip Saponaro, Anita Schwartz
Students: Hening Cui
Project Description
Using existing image datasets from different microscopy platforms, we would like to cross validate and, if needed, retrain the deep-learning model (U-Net CNN) used by DeepXScope for 3D segmentation. Cross validation and retraining of the model will be performed using real data as well as virtual data (fungal infection on host tissue is created by computer simulation). With a validated pipeline, DeepXScope will then be applied to available datasets to generate associated output data for downstream analysis and biological interpretation.Background: Plant diseases limit the production of crop plants worldwide. Durable forms of natural resistance (genetically determined) are an environmentally friendly and sustainable solution for disease control, but the biology of durable resistance is poorly understood, particularly at the microscale where the pathogens are visible. To better understand pathogenesis, we developed DeepXScope (https://github.com/drmaize/compvision): a deep-learning facilitated pipeline for segmenting 3D microscopy data and quantifying features of host-pathogen interactions. Working in maize (corn) on a fungus causing prior epidemics, DeepXScope is being used to show how macroscopic disease outcomes arise from microscopic events during pathogenesis.
Additional Resources
Launch Presentation:Wrap Presentation: 6 months