Unsupervised learning of topologically ordered phases of matter
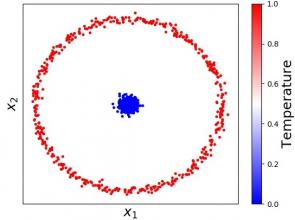
Project Information
gpu, machine-learning, quantum-mechanicsProject Status: Complete
Project Region: Northeast
Submitted By: Northeast Cyberteam
Project Email: cherdman@middlebury.edu
Project Institution: Middlebury College
Anchor Institution: NE-University of Vermont
Mentors: Adrian Del Maestro
Students: Gebremedhin Dagnew
Project Description
Identifying and distinguishing new phases of matter is a key challenge in condensed matter physics physics. Recent work has demonstrated that machine learning techniques can be used to identify phases of matter with a broken symmetry. Certain quantum phases of matter, such as spin liquids, have a topological order that doesn’t break conventional symmetries, and thus are harder to identify. However, supervised machine learning techniques have recently been successfully used to identify topologically ordered phases. This project seeks to extend this work to use unsupervised learning algorithms to identify topological phases of matter. In particular, this project will focus on applying dimensional reduction algorithms to study topologically ordered phases of matter. These algorithms will be implemented to take advantage of GPUs and deployed on an HPC cluster.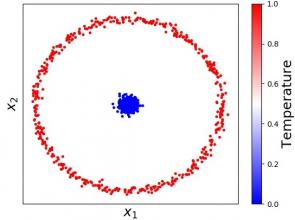
Project Information
gpu, machine-learning, quantum-mechanicsProject Status: Complete
Project Region: Northeast
Submitted By: Northeast Cyberteam
Project Email: cherdman@middlebury.edu
Project Institution: Middlebury College
Anchor Institution: NE-University of Vermont
Mentors: Adrian Del Maestro
Students: Gebremedhin Dagnew