Gravitational-wave Inference with Waveform Uncertainty
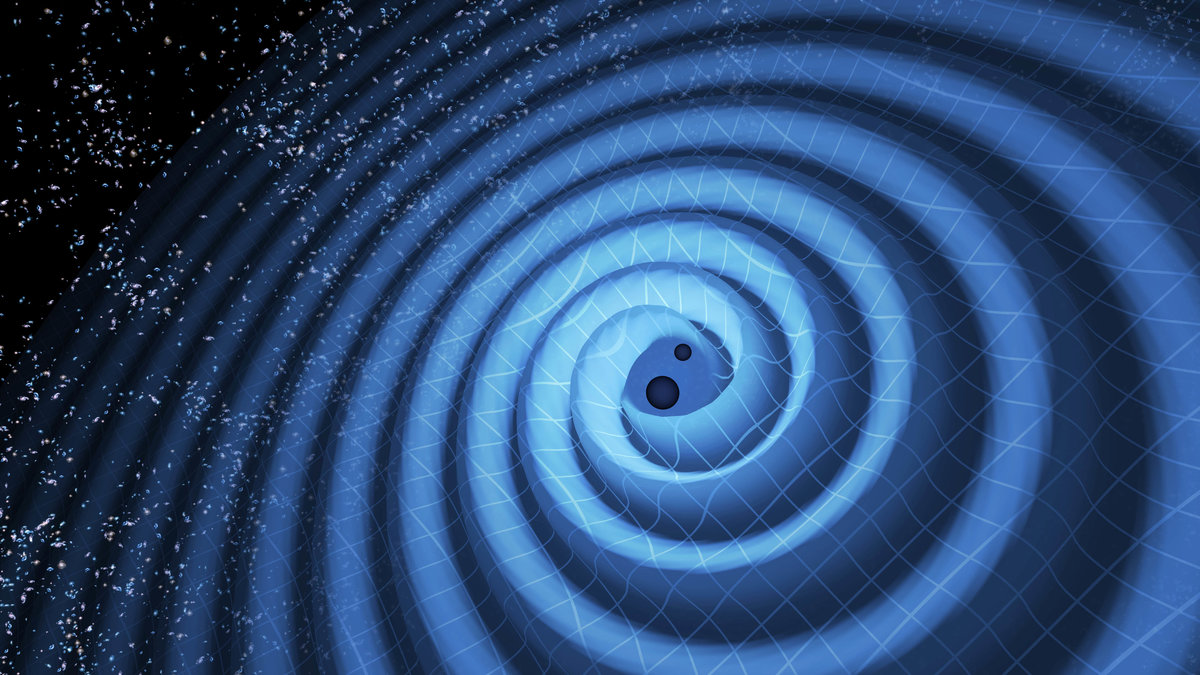
Project Information
astrophysics, benchmarking, deep-learning, gravitational-waves, machine-learningProject Status: Complete
Project Region: CAREERS
Submitted By: Gaurav Khanna
Project Email: mpuerrer@uri.edu
Project Institution: University of Rhode Island -- Center for Computational Research
Anchor Institution: CR-University of Rhode Island
Mentors: Gaurav Khanna
Students: Ritesh Bachhar
Project Description
Models of gravitational waves (GW) emitted from merging black hole binaries are based on numerical data and modeling assumptions which introduces sources of error. When performing Bayesian inference of the binary parameters from a GW signal these models are used to compute the posterior distribution with a sampling algorithm. This project aims at including marginalization over waveform uncertainty at the inference stage. The resulting posterior distribution is expected to peak closer to the true signal parameters at the cost of a slight broadening of the distribution, trading precision for improved accuracy.In this project the student will adapt computational inference workflows to execute on URI’s UNITY cluster and perform extensive testing using real and synthetic GW events while marginalizing over internal degrees of freedom of an effective-one-body waveform model. If time permits, the student will use the developed workflow to compare two methodologies: (i) a probabilistic Gaussian process regression (GPR) waveform model using a standard stochastic sampler, and (ii) using the novel technique of neural posterior estimation on training set data augmented with waveform uncertainties, leveraging the deep learning-based Dingo inference code.
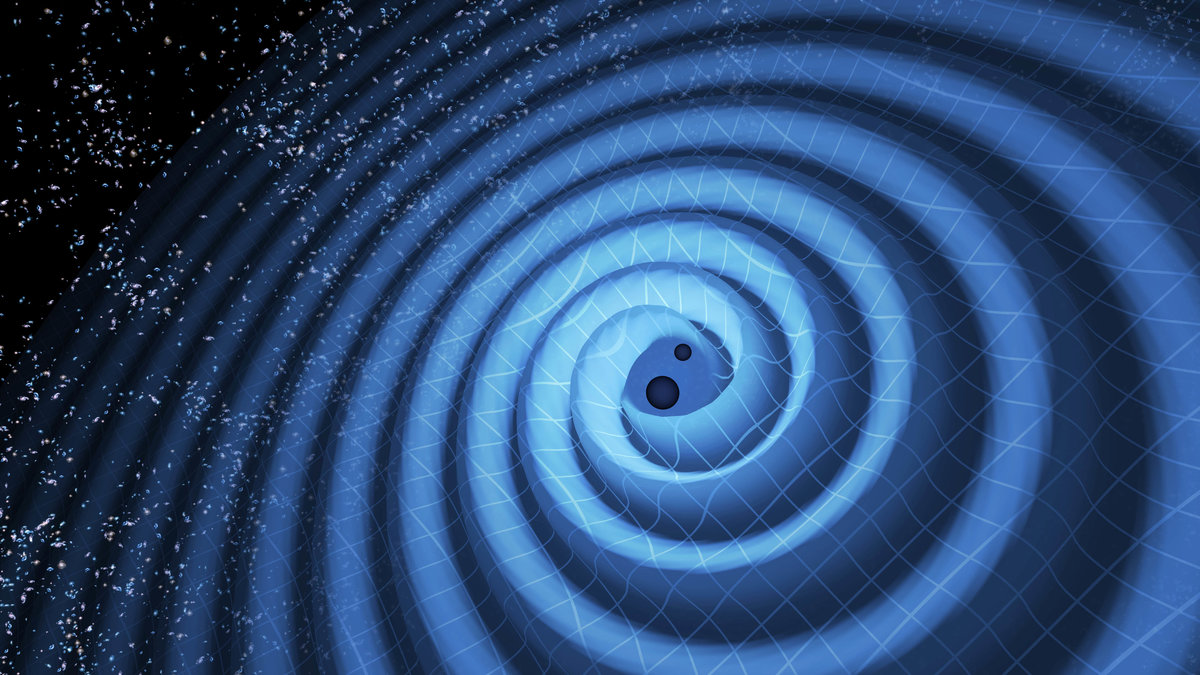
Project Information
astrophysics, benchmarking, deep-learning, gravitational-waves, machine-learningProject Status: Complete
Project Region: CAREERS
Submitted By: Gaurav Khanna
Project Email: mpuerrer@uri.edu
Project Institution: University of Rhode Island -- Center for Computational Research
Anchor Institution: CR-University of Rhode Island
Mentors: Gaurav Khanna
Students: Ritesh Bachhar
Project Description
Models of gravitational waves (GW) emitted from merging black hole binaries are based on numerical data and modeling assumptions which introduces sources of error. When performing Bayesian inference of the binary parameters from a GW signal these models are used to compute the posterior distribution with a sampling algorithm. This project aims at including marginalization over waveform uncertainty at the inference stage. The resulting posterior distribution is expected to peak closer to the true signal parameters at the cost of a slight broadening of the distribution, trading precision for improved accuracy.In this project the student will adapt computational inference workflows to execute on URI’s UNITY cluster and perform extensive testing using real and synthetic GW events while marginalizing over internal degrees of freedom of an effective-one-body waveform model. If time permits, the student will use the developed workflow to compare two methodologies: (i) a probabilistic Gaussian process regression (GPR) waveform model using a standard stochastic sampler, and (ii) using the novel technique of neural posterior estimation on training set data augmented with waveform uncertainties, leveraging the deep learning-based Dingo inference code.